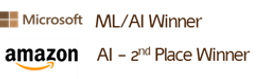
Our AI Story:
It all started with a strawberry, TRIZ thesis and navigating complexity!
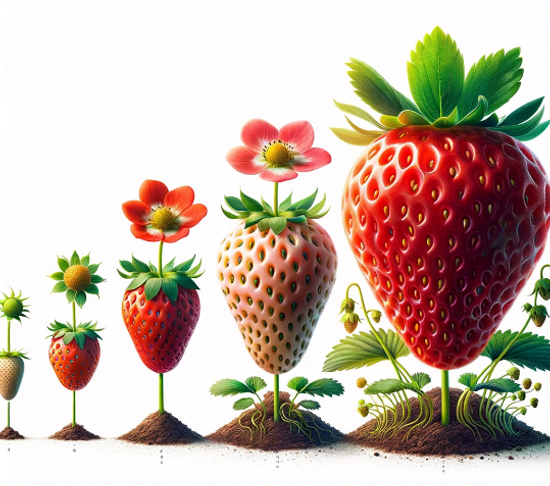
Every fruit, every vegetable, and everything in nature carries its own story, defined by variety, size, and growth stage. Our mission was to decode this complexity into actionable data. Our journey began with specialty crops; our technology is now for ALL commodities. We knew long ago AI depended on reliable, thoroughly researched, embedded, trusted, verified, and contained data. Our patent on Supply Chain Intelligence – Methods and Systems confirms our thesis.
Artificial intelligence (AI) is revolutionizing industries, including the food supply chain. AI helps companies make better decisions by using machine learning, a type of AI that allows computers to learn from data and improve over time. agtools® proprietary data and data collection has developed a cutting-edge technology that significantly enhances this learning process, especially in specialty crops such as fruits and vegetables. However, it is versatile enough to handle any commodity.

Data Collection
The first step in machine learning is collecting data. In the data collection phase, information about market conditions, commodity prices, and supply locations is gathered from various sources. This step involves not only the accumulation of raw data but also the initial review to ensure the data’s validity and relevance to the commodity. For instance, data about seasonal fruit supply from certain regions give sourcing managers, buyers, and merchandisers information with which to plan promotions and product display space allocation, among other things.
Agtools technology gathers vast amounts of market reference data going back over 25 years—this includes everything from the prices of the commodity in different seasons to the volume shipped from producing regions. Since accurate data is crucial for AI, the quality of this initial collection step is vital.
Data Cleaning and Curation
Once data is collected, it may contain errors, duplicates, or irrelevant details that need to be corrected or removed. Data curation further involves organizing this cleaned data into a structured format suitable for analysis. This process ensures the data is correct, coherent, and easily accessible.
Agtools automated technology, combined with assisted processes, cleans this data, removing inaccuracies and organizing it to be useful. For example, if the data for a particular commodity breaks a pattern, our systems detect that apparent anomaly, and it is referred to analysts to review the source, the quality of the input, and any potential correction necessary. On occasions, team members contact the source, adding a superbly important layer of revision that distinguishes Agtools data from other sources on the commodities it tracks.

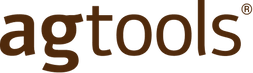
Auditing and Contextualization
Auditing involves thoroughly reviewing the data to validate its accuracy and consistency over time. Contextualization by Agtools adds a layer of domain-specific knowledge, enhancing the data with insights specific to the food supply chain, such as regional production factors, economic impacts on pricing, or the effect of climate on quality in the context of each plant’s development phase.
Based on the company’s deep expertise in the food supply chain, Agtools audits and enriches the data, embedding it with contextual insights crucial for accurate interpretation. This ensures the data is correct and enriched with meaningful insights that reflect real-world complexities.
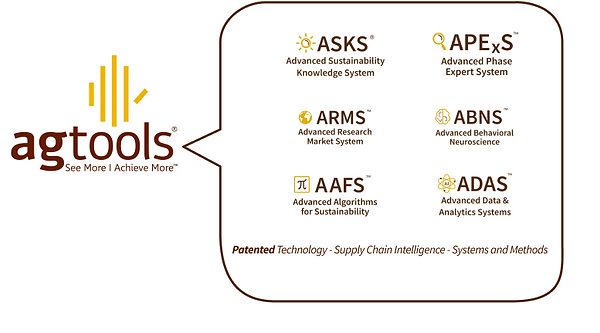.png)
Generating Insights
Finally, the cleaned, curated, audited, and contextualized data is ready for machine learning applications. The AI uses this prepared data to identify patterns and make predictions. For instance, it might predict that the price of a commodity will rise due to a forecasted drought in key growing areas. Companies can use these insights to make informed decisions, like stocking up on the commodity before sourcing is impacted and prices react accordingly.
Agtools technology is pivotal at every step of this process. It ensures the data is accurate, understandable, and ready for AI to use. This leads to smarter decisions in the supply chain, optimizing everything from pricing strategies to inventory management. By enabling more accurate machine learning analyses, our client is not just participating in the AI revolution—they are leading it in the food supply industry.
Process Efficiency
The data preparation phase of Data Analytics requires considerable resources. Some estimates place data preparation efforts as high as 80% of the cost and resources necessary to feed machine learning models and artificial intelligence projects. Agtools delivers clean and prepared data to its clients.
Whether the data is to be used as a standalone set or cross-referenced to first-party data for enhanced insights, companies save considerable time and resources – human and financial – by incorporating Agtools into their machine learning and artificial intelligence journey.

